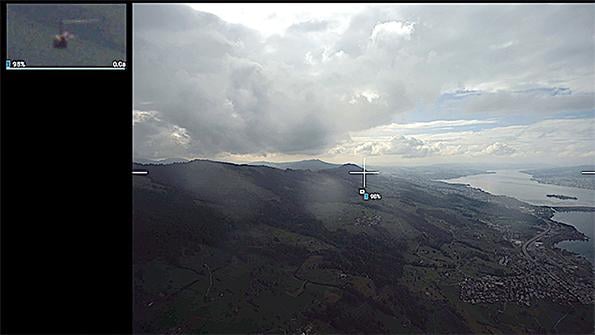
While commercial use of electric air taxis is expected to begin by 2024-25, Europe’s aviation regulator believes it will take at least five more years to enable autonomous passenger transport, the holy grail for ubiquitous and affordable urban air mobility.
While autonomy will take longer, it is expected to begin with small aircraft. “It’s easier to start to deal with autonomous passenger transport with air taxi vehicles because we are looking at smaller aircraft and a smaller number of passengers,” says European Union Aviation Safety Agency (EASA) Executive Director Patrick Ky.
- AI for pilot assistance expected by 2025
- Automated air taxis likely beyond 2030
“The vehicles we are certifying are piloted air taxis. But the companies developing them have clearly stated that it’s a first step, and the second step is expected to be unmanned air taxis,” he says. “We have started to work with them on this, but we have not gone too far because it requires a lot of thinking on how you want to deal with this autonomy concept. Is it a full autonomy? Is it remotely piloted? How do you interact with air traffic management? We have just started to look into it,” Ky says.
Artificial intelligence (AI) is expected to be a key enabler of autonomy, and here EASA is moving ahead, issuing its first usable guidance on requirements for safety-related machine-learning (ML) applications. The concept paper is open for public consultation through June 30.
Release of the guidance document follows the publication of EASA’s AI Roadmap V1.0 in February 2020 and builds on work with industry to develop design assurance concepts for ML neural networks in safety-critical applications. EASA has completed two joint projects with Swiss AI startup Daedalean on visual landing guidance and visual traffic-detection systems using machine learning.
The AI Roadmap established three levels of AI/ML applications, and the guidance document presents a first set of objectives for Level 1—human augmentation and assistance. Level 2 covers human-AI collaboration, and Level 3 involves more autonomous (3A) and fully autonomous (3B) AI.
The AI levels are aligned with the high-level functions of increasing automation: information acquisition and analysis for Level 1; decision-making and action implementation for Levels 2 and 3. Level 2 is when the human is monitoring the system and can intervene in every decision it makes or action it takes.
The more autonomous Level 3A is overridable, meaning the human is supervising the system and not involved in every decision or action but can override when necessary for safety or security. Level 3B is nonoverridable, meaning the human is out of the loop and cannot override the system.
“It’s important to note that, for all of these levels, full oversight of the design phase is a mandatory prerequisite,” says Guillaume Soudain, EASA senior software expert. “None of these cases will fully escape human oversight. But entering into 3B also will require extremely powerful safety and security analyses and ethics-based assessment.”
The concept paper has two goals. The first is to allow applicants using ML techniques in projects to have early visibility into possible EASA expectations for the implementation of AI. “The second is to establish a baseline for AI Level 1 applications. That will be further refined when we develop the Level 2 and Level 3 applications,” Soudain says.
The document is a first step in a two-phase process beginning with guidance development and leading to rulemaking for AI applications. The initial concept paper on Level 1 AI/ML will be followed by guidance documents for Level 2 in 2022 and Level 3 in 2024. Final rules are planned to follow by 2026 for Levels 1 and 2 and by 2028 for Level 3.
EASA expects to approve its first AI/ML applications in 2025, and its road map foresees the potential—enabled by the technology and based in industry input—for large commercial aircraft operations to be single-pilot by 2030 and fully autonomous by 2035.
The first guidance document is limited to nonadaptive supervised learning for safety-related applications. “Supervised learning is a technique that supervises how the datasets are labeled. Nonadaptive means that the model is frozen, so it’s not continuously learning in operations,” says Soudain.
The driving concept behind EASA’s approach is AI trustworthiness. “This can be summarized by four building blocks,” he said. “The first is AI trustworthiness analysis. It’s an essential gate that aims at characterizing the AI application.”
The first step in characterizing an AI application is to identify the high-level functions to be performed by the system and the overall concept of operation, Soudain says. This involves identifying the potential operational scenarios and performing a human-centric analysis of all users that interact with the system.
AI trustworthiness analysis encompasses proven safety and security assessments that have been adapted to machine learning. “This AI trustworthiness analysis block also triggers an ethics-based assessment, which is a translation from the European Commission’s ethical guidelines into a framework practicable for our aviation industry,” he says. These high-level guidelines cover societal concerns such as accountability, oversight, privacy and transparency.
The next building block is learning assurance, an innovation developed by EASA in collaboration with industry. “The fundamental question behind this building block is: How do we ensure confidence that machine-learning applications will behave as intended?” Soudain says.
The standard V-shaped development assurance process has been adapted and augmented with a W-shaped learning assurance process that begins with data management and puts a specific focus on the completeness and representativeness of the data used to train the ML algorithm.
After this comes the learning process, building a trained model and verifying its performance. The next step is to transform the trained model onto the inference platform—the hardware and software that runs the algorithm on the aircraft—in a way that does not adversely affect its behavior.
“To close the data management and learning processes, an independent verification step is foreseen at the end of the W-shaped process as a final gate before entering a more traditional requirements-based verification at the subsystem or system level,” Soudain says.
The next building block is AI explainability. “The explainability block is paramount for the acceptability of AI in aviation. It is designed with the objective of maintaining the trust of all stakeholders involved over the whole life cycle of the AI-based system,” says EASA expert Francois Triboulet.
Stakeholders include flight crews, air traffic controllers and maintainers as well as developers, certification authorities and safety investigators. “All these end users express their needs in terms of trust, level of confidence and ease and effectiveness of collaboration with the AI-based system. Explainability has to bring solutions to all these different needs,” Triboulet says.
Aspects of AI explainability include intuitive human-machine interfaces, real-time monitoring to ensure the AI-based system stays within the defined boundaries of its design operating domain and recording and processing of operations data to detect deviations from expected behavior.
The final part of EASA’s framework is the AI safety risk-mitigation block. “[This] is an anticipation of the difficulty in some cases of fulfilling the learning assurance and/or explainability provisions,” says Soudain. For now, this step is about assessing and mitigating any residual risk, “[but] this block is meant to be evolving as the rest of the guidance is developed,” he says.
Comments
Especially noted the sentence, "EASA...foresees the potential—enabled by the technology and based in industry input—for large commercial aircraft operations to be single-pilot by 2030 and fully autonomous by 2035."