This article is published in Aviation Week & Space Technology and is free to read until May 16, 2024. If you want to read more articles from this publication, please click the link to subscribe.
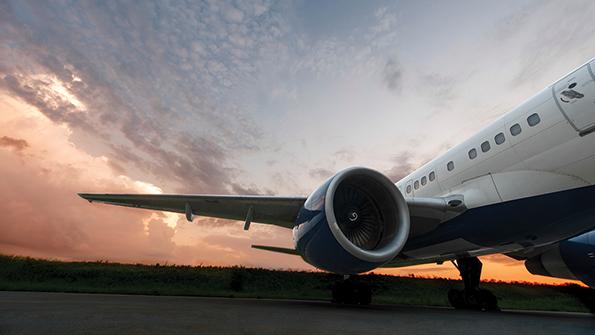
Scrap forecasting accuracy has improved at Delta TechOps through better use of data.
“Technology now just allows you to make a bad mistake faster,” Mike McBride, Delta TechOps’ vice president for maintenance operations, observed at the Aviation Week Network’s MRO Americas conference on April 10 as he stressed the importance of careful advance groundwork before migrating to new technologies. The insights were shared during a panel on artificial intelligence.
“When we started to get into more advanced predictive [technologies] and started to really think about how to employ AI, the projects early on that we did, two-thirds of the work was on the data itself and trying to make sure that we had the proper setup and the proper platform and had the data streams that weren’t corrupt,” McBride said. “The work that goes on behind the scenes before the sexy reveal of the ‘new latest thing’ is two-thirds of the battle.”
- AI is unlocking cost savings and efficiencies
- Cautious approaches to generative AI are key, companies say
Scrap prediction is one area of AI opportunity at Delta TechOps: Teams strategized about how to infuse information from engines in operation into its prediction models. With more control earlier in the process, costs can be reined in, and its scrap forecasting accuracy has improved considerably.
“That’s where the AI started to come in,” McBride explained. “They started to just get creative about the parameters that they were going to use to assess scrap rates. Our forecasting, for one thing, the window extended—you’re 3-6 months in the old modeling world, and now we’re 12-18 [months] to working further out than that. We’re really watching the fleet at a different level.”
Proof-of-concept testing utilizing the old and new systems on Pratt & Whitney’s PW2000 engines demonstrated considerable cost savings, McBride noted. “We’ve had a lot of fun with it and learned a lot, actually, and [we are] looking forward to where we go with it next,” he said.
The emergence and evolution of generative AI—which is capable of creating new output based on what it learns—is the next chapter. Companies described cautious approaches as they look to unlock its full potential through the right people, processes, data and tools.
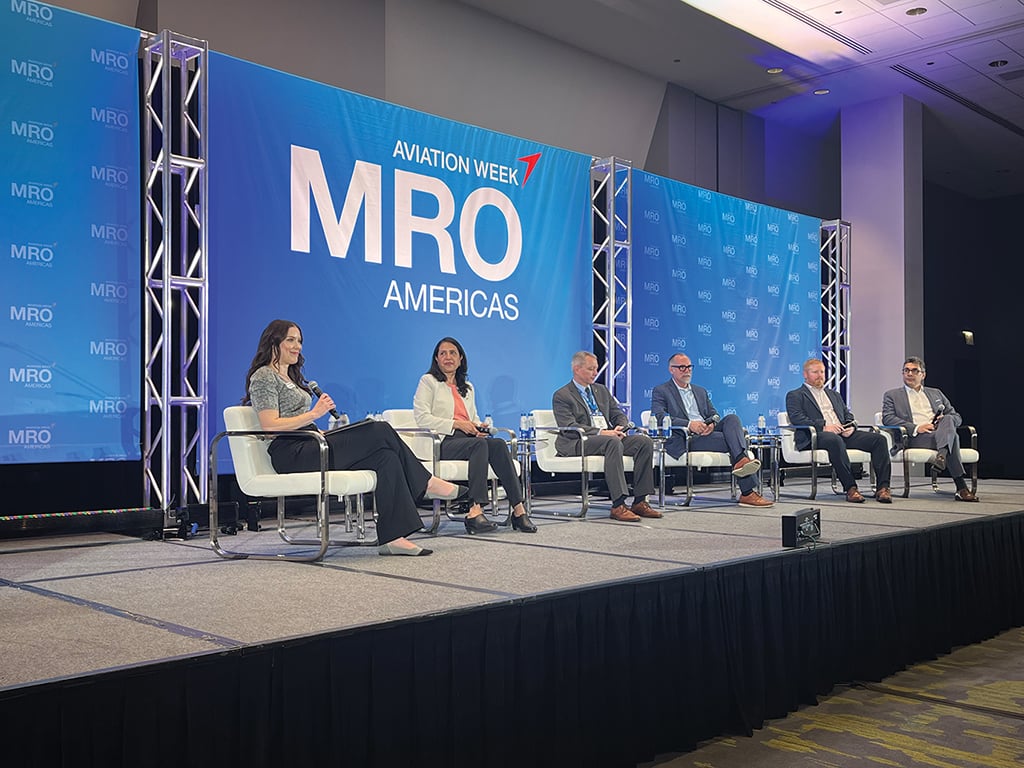
A “responsible AI program” has been formed at United Airlines for visibility into all generative AI use cases across the airline, Director of Digital Fleet Management Luke Meminger said.
United’s initial applications of generative AI have begun on the customer side, with teams using it as a co-pilot to generate messaging around delays or other outward-facing communications. “There’s some learnings out of that, on how you ask questions to gen AI and get answers,” Meminger observed. “Depending on how nice you are when you ask the question, [you] get a different answer back.”
Another “lighter-weight” implementation the carrier described is the ability to interact with documents. The airline applied a large language model (LLM)—a type of generative AI—against its recent pilot contract agreement, facilitating queries into the more than 2,000-page document.
Consultancy McKinsey & Co. described several ways of looking at how to implement generative AI technologies—whether through an open-source ChatGPT-type solution, a build-it-yourself “maker model” or a somewhat in-between “shaper” model. Ensuring access to correct data is key; otherwise, “it will reinforce itself hearing the wrong thing,” explained expert associate partner Dave Marcontell.
“We believe that answer is this hybrid of where you leverage some of the algorithms from the open-source piece and apply that to protected proprietary datasets that exist within the airlines and the OEMs that are a much more controlled environment,” he said. “In our view, it starts there. It’s that hybrid of datasets between the airlines and the OEMs.”
At Boeing, an LLM was developed from scratch. With access to considerable historical data, it built its own aviation-domain LLM using maintenance records, maintenance logs, reports and so forth to derive intelligence from those sources.
Naina Dhareshwar, chief technology officer for Boeing Digital Aviation Solutions, led with an example from the predictive side.
“Because of the intelligence that we’ve built, we are able to say what part will fail and how often it will fail and how you position the parts ahead of time, based on all the predictive capabilities,” she said.
On the generative AI side, the OEM is starting with internal use.
From a solutions perspective, Dhareshwar sees generative AI not as a competing with predictive maintenance but rather augmenting it. The technology has the ability to “find the nuggets” in unstructured data and use it in a governed manner to enhance predictive maintenance capability, which Dhareshwar described as Boeing’s current focus: “It’s no longer two plus two equal to four, but it’s two plus two equal to a power of four.”